概率图详解¶
概述¶
probscale.probplot
函数允许你做以下几件事:
- 创建百分位、分位数或概率图。
- 将概率刻度放在任一轴上。
- 为概率刻度指定任意分布。
- 在线性概率或对数概率空间中绘制最佳拟合线。
- 以你想要的任何方式计算数据的绘图位置。
- 在 seaborn
FacetGrids
上使用概率轴
我们将在本教程中介绍所有这些选项。
%matplotlib inline
import warnings
warnings.simplefilter('ignore')
import numpy
from matplotlib import pyplot
import seaborn
import probscale
clear_bkgd = {'axes.facecolor':'none', 'figure.facecolor':'none'}
seaborn.set(style='ticks', context='talk', color_codes=True, rc=clear_bkgd)
# load up some example data from the seaborn package
tips = seaborn.load_dataset("tips")
iris = seaborn.load_dataset("iris")
不同的绘图类型¶
一般来说,有三种绘图类型
- 百分位图,又名 P-P 图
- 分位数图,又名 Q-Q 图
- 概率图,又名 Prob 图
百分位图¶
百分位图是最简单的图。你只需将数据绘制在其绘图位置上。绘图位置显示在线性刻度上,但可以根据需要缩放数据。
如果你从头开始做,它会看起来像这样
position, bill = probscale.plot_pos(tips['total_bill'])
position *= 100
fig, ax = pyplot.subplots(figsize=(6, 3))
ax.plot(position, bill, marker='.', linestyle='none', label='Bill amount')
ax.set_xlabel('Percentile')
ax.set_ylabel('Total Bill (USD)')
ax.set_yscale('log')
ax.set_ylim(bottom=1, top=100)
seaborn.despine()
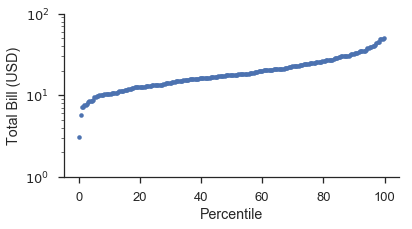
使用 probplot
函数和 plottype='pp'
,它会变成
fig, ax = pyplot.subplots(figsize=(6, 3))
fig = probscale.probplot(tips['total_bill'], ax=ax, plottype='pp', datascale='log',
problabel='Percentile', datalabel='Total Bill (USD)',
scatter_kws=dict(marker='.', linestyle='none', label='Bill Amount'))
ax.set_ylim(bottom=1, top=100)
seaborn.despine()
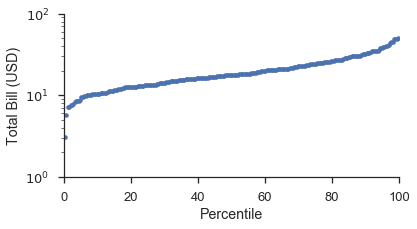
分位数图¶
分位数图类似于概率图。主要区别在于,绘图位置会根据概率分布转换为分位数或 \(Z\) 分数。默认分布是标准正态分布。使用不同分布将在下文进一步介绍。
使用与上面相同的数据集,我们来制作一个分位数图。和上面一样,我们将从头开始做,然后使用 probplot
。
from scipy import stats
position, bill = probscale.plot_pos(tips['total_bill'])
quantile = stats.norm.ppf(position)
fig, ax = pyplot.subplots(figsize=(6, 3))
ax.plot(quantile, bill, marker='.', linestyle='none', label='Bill amount')
ax.set_xlabel('Normal Quantiles')
ax.set_ylabel('Total Bill (USD)')
ax.set_yscale('log')
ax.set_ylim(bottom=1, top=100)
seaborn.despine()
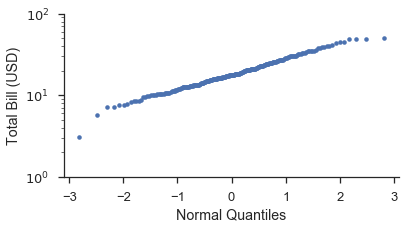
使用 probplot
fig, ax = pyplot.subplots(figsize=(6, 3))
fig = probscale.probplot(tips['total_bill'], ax=ax, plottype='qq', datascale='log',
problabel='Standard Normal Quantiles', datalabel='Total Bill (USD)',
scatter_kws=dict(marker='.', linestyle='none', label='Bill Amount'))
ax.set_ylim(bottom=1, top=100)
seaborn.despine()
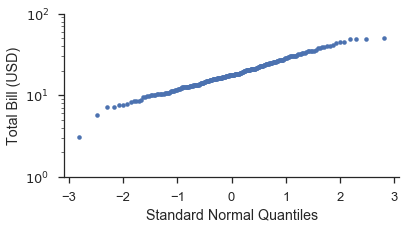
你会注意到,在 Q-Q 图上,数据的形状比在 P-P 图上更直。这是由于将绘图位置转换为分布的分位数时发生的转换所致。下面的图希望能更清楚地说明这一点。此外,我们将展示如何使用 probax
选项来翻转绘图,以便 P-P/Q-Q/概率轴位于 y 轴上。
fig, (ax1, ax2) = pyplot.subplots(figsize=(6, 6), ncols=2, sharex=True)
markers = dict(marker='.', linestyle='none', label='Bill Amount')
fig = probscale.probplot(tips['total_bill'], ax=ax1, plottype='pp', probax='y',
datascale='log', problabel='Percentiles',
datalabel='Total Bill (USD)', scatter_kws=markers)
fig = probscale.probplot(tips['total_bill'], ax=ax2, plottype='qq', probax='y',
datascale='log', problabel='Standard Normal Quantiles',
datalabel='Total Bill (USD)', scatter_kws=markers)
ax1.set_xlim(left=1, right=100)
fig.tight_layout()
seaborn.despine()
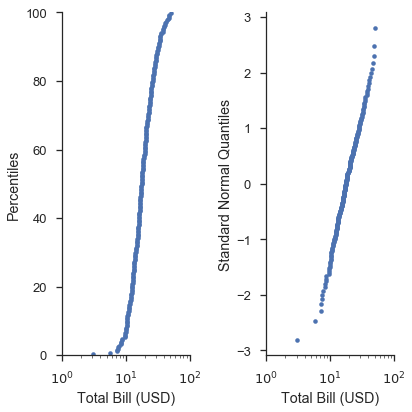
在 P-P 图和简单的 Q-Q 图的情况下,与编写原始 matplotlib 命令相比,probplot
函数没有提供太多的便利。但是,当你开始制作概率图并使用更高级的选项时,这种情况就会改变。
概率图¶
从视觉上看,概率和分位数刻度上的曲线应该相同。不同之处在于,轴刻度线是根据非超出的概率放置和标记的,而不是分布的更抽象的分位数。
毫不奇怪,图片能更好地解释这一点。让我们以上一个图为基础构建
fig, (ax1, ax2, ax3) = pyplot.subplots(figsize=(9, 6), ncols=3, sharex=True)
common_opts = dict(
probax='y',
datascale='log',
datalabel='Total Bill (USD)',
scatter_kws=dict(marker='.', linestyle='none')
)
fig = probscale.probplot(tips['total_bill'], ax=ax1, plottype='pp',
problabel='Percentiles', **common_opts)
fig = probscale.probplot(tips['total_bill'], ax=ax2, plottype='qq',
problabel='Standard Normal Quantiles', **common_opts)
fig = probscale.probplot(tips['total_bill'], ax=ax3, plottype='prob',
problabel='Standard Normal Probabilities', **common_opts)
ax3.set_xlim(left=1, right=100)
ax3.set_ylim(bottom=0.13, top=99.87)
fig.tight_layout()
seaborn.despine()
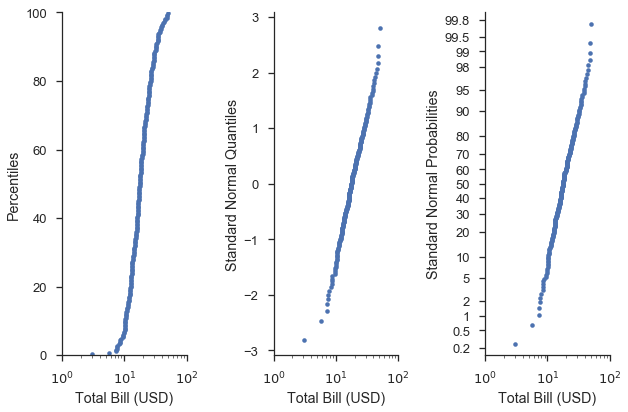
从视觉上看,最右侧图上的曲线形状是相同的。不同之处在于,y 轴刻度线和标签更“人性化”。
换句话说,概率(右)轴使我们能够轻松找到例如百分位(左)轴上的第 75 个百分位数,并说明数据如何很好地拟合给定的分布,如分位数(中间)轴所示。
为刻度使用不同的分布¶
使用分位数或概率刻度时,可以将 scipy.stats
模块中的分布传递给 probplot
函数。如果没有为 dist
参数提供分布,则使用标准正态分布。
common_opts = dict(
plottype='prob',
probax='y',
datascale='log',
datalabel='Total Bill (USD)',
scatter_kws=dict(marker='+', linestyle='none', mew=1)
)
alpha = stats.alpha(10)
beta = stats.beta(6, 3)
fig, (ax1, ax2, ax3) = pyplot.subplots(figsize=(9, 6), ncols=3, sharex=True)
fig = probscale.probplot(tips['total_bill'], ax=ax1, dist=alpha,
problabel='Alpha(10) Probabilities', **common_opts)
fig = probscale.probplot(tips['total_bill'], ax=ax2, dist=beta,
problabel='Beta(6, 1) Probabilities', **common_opts)
fig = probscale.probplot(tips['total_bill'], ax=ax3, dist=None,
problabel='Standard Normal Probabilities', **common_opts)
ax3.set_xlim(left=1, right=100)
for ax in [ax1, ax2, ax3]:
ax.set_ylim(bottom=0.2, top=99.8)
seaborn.despine()
fig.tight_layout()
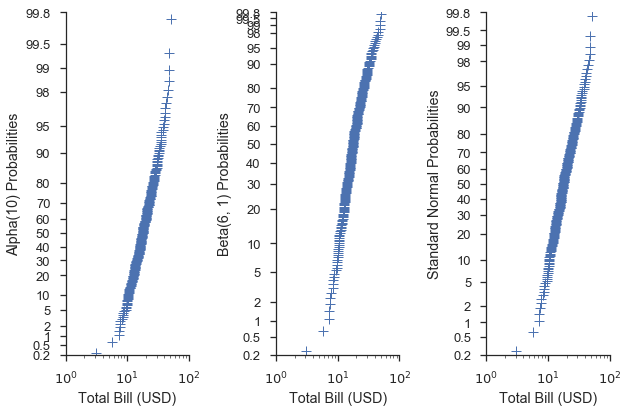
这也适用于 QQ 刻度
common_opts = dict(
plottype='qq',
probax='y',
datascale='log',
datalabel='Total Bill (USD)',
scatter_kws=dict(marker='+', linestyle='none', mew=1)
)
alpha = stats.alpha(10)
beta = stats.beta(6, 3)
fig, (ax1, ax2, ax3) = pyplot.subplots(figsize=(9, 6), ncols=3, sharex=True)
fig = probscale.probplot(tips['total_bill'], ax=ax1, dist=alpha,
problabel='Alpha(10) Quantiles', **common_opts)
fig = probscale.probplot(tips['total_bill'], ax=ax2, dist=beta,
problabel='Beta(6, 3) Quantiles', **common_opts)
fig = probscale.probplot(tips['total_bill'], ax=ax3, dist=None,
problabel='Standard Normal Quantiles', **common_opts)
ax1.set_xlim(left=1, right=100)
seaborn.despine()
fig.tight_layout()
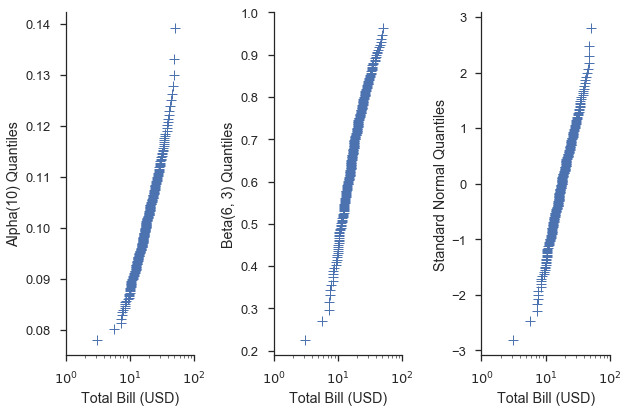
在分位数刻度中使用特定的分布可以让我们了解数据如何很好地拟合该分布。例如,假设我们有一个预感,我们的数据集中的 total_bill
列的值是正态分布的,并且它们的平均值和标准差分别为 19.8 和 8.9。我们可以通过创建一个具有这些参数的 scipy.stat.norm
分布,并在 Q-Q 图中使用该分布来进行研究。
def equality_line(ax, label=None):
limits = [
numpy.min([ax.get_xlim(), ax.get_ylim()]),
numpy.max([ax.get_xlim(), ax.get_ylim()]),
]
ax.set_xlim(limits)
ax.set_ylim(limits)
ax.plot(limits, limits, 'k-', alpha=0.75, zorder=0, label=label)
norm = stats.norm(loc=21, scale=8)
fig, ax = pyplot.subplots(figsize=(5, 5))
ax.set_aspect('equal')
common_opts = dict(
plottype='qq',
probax='x',
problabel='Theoretical Quantiles',
datalabel='Emperical Quantiles',
scatter_kws=dict(label='Bill amounts')
)
fig = probscale.probplot(tips['total_bill'], ax=ax, dist=norm, **common_opts)
equality_line(ax, label='Guessed Normal Distribution')
ax.legend(loc='lower right')
seaborn.despine()
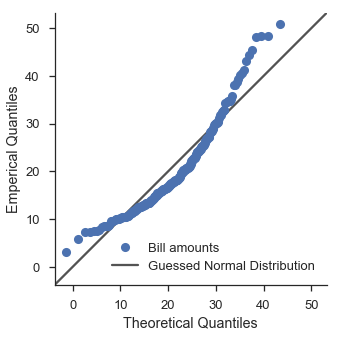
嗯。看起来不太好。让我们使用 scipy 的拟合功能来尝试对数正态分布。
lognorm_params = stats.lognorm.fit(tips['total_bill'], floc=0)
lognorm = stats.lognorm(*lognorm_params)
fig, ax = pyplot.subplots(figsize=(5, 5))
ax.set_aspect('equal')
fig = probscale.probplot(tips['total_bill'], ax=ax, dist=lognorm, **common_opts)
equality_line(ax, label='Fit Lognormal Distribution')
ax.legend(loc='lower right')
seaborn.despine()
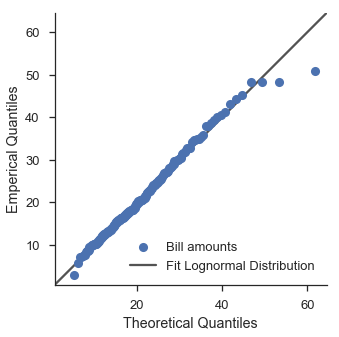
稍微好一点了。
找到最佳分布留给读者练习。
最佳拟合线¶
在概率图中添加最佳拟合线可以帮助你了解数据集是否可以用分布来描述。
这只需在 probplot
中使用 bestfit=True
选项即可。在后台,probplot
会根据绘图类型和数据轴的刻度(通过 datascale
控制)转换馈送到回归的 x 和 y 数据。
可以使用 line_kws
参数来控制直线的视觉属性。如果你想标记最佳拟合线,则可以在此处指定其标签。
简单示例¶
最简单的情况是具有线性数据轴的 P-P 图
fig, ax = pyplot.subplots(figsize=(6, 3))
fig = probscale.probplot(tips['total_bill'], ax=ax, plottype='pp', bestfit=True,
problabel='Percentile', datalabel='Total Bill (USD)',
scatter_kws=dict(label='Bill Amount'),
line_kws=dict(label='Best-fit line'))
ax.legend(loc='upper left')
seaborn.despine()
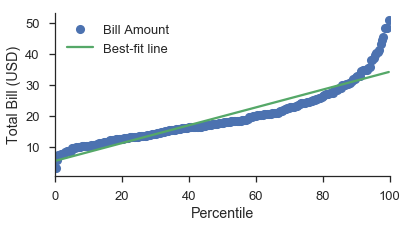
最不简单的情况是具有对数缩放数据轴的概率图。
正如自定义分布分位数图部分所建议的那样,使用对数正态数据刻度的正态概率刻度提供了不错的拟合(从视觉上讲)。
请注意,你仍然可以将概率刻度放在 x 轴或 y 轴上。
fig, ax = pyplot.subplots(figsize=(4, 6))
fig = probscale.probplot(tips['total_bill'], ax=ax, plottype='prob', probax='y', bestfit=True,
datascale='log', problabel='Probabilities', datalabel='Total Bill (USD)',
scatter_kws=dict(label='Bill Amount'),
line_kws=dict(label='Best-fit line'))
ax.legend(loc='upper left')
ax.set_ylim(bottom=0.1, top=99.9)
ax.set_xlim(left=1, right=100)
seaborn.despine()
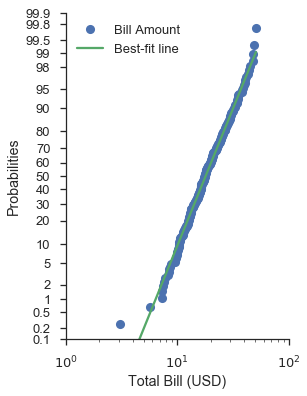
自举置信区间¶
无论绘图的刻度如何(线性、对数或概率),都可以在最佳拟合线周围添加自举置信区间。只需将 estimate_ci=True
选项与 bestfit=True
一起使用
N = 15
numpy.random.seed(0)
x = numpy.random.normal(size=N) + numpy.random.uniform(size=N)
fig, ax = pyplot.subplots(figsize=(8, 4))
fig = probscale.probplot(x, ax=ax, bestfit=True, estimate_ci=True,
line_kws={'label': 'BF Line', 'color': 'b'},
scatter_kws={'label': 'Observations'},
problabel='Probability (%)')
ax.legend(loc='lower right')
ax.set_ylim(bottom=-2, top=4)
seaborn.despine(fig)
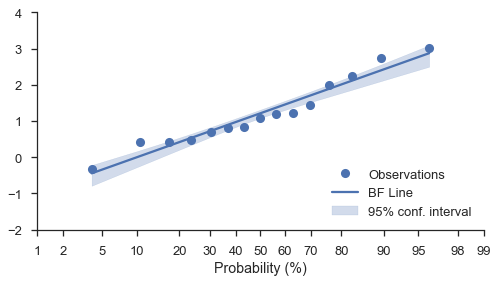
调整绘图位置¶
probplot
函数调用 viz.plot_plos()
函数来计算每个数据集的绘图位置。
你应该阅读该函数的文档字符串以获取更详细的信息。但是,高级概述是,你可以在绘图位置计算中调整几个参数(alpha
和 beta
)。
可以通过 postype
参数选择最常见的值。
这些通过 probplot 中的 pp_kws 参数控制,并在下一个教程中更详细地讨论。
common_opts = dict(
plottype='prob',
probax='x',
datalabel='Data',
)
numpy.random.seed(0)
x = numpy.random.normal(size=15)
fig, (ax1, ax2, ax3) = pyplot.subplots(figsize=(6, 6), nrows=3,
sharey=True, sharex=True)
fig = probscale.probplot(x, ax=ax1, problabel='Cunnuane (default) plotting positions',
**common_opts)
fig = probscale.probplot(x, ax=ax2, problabel='Weibull plotting positions',
pp_kws=dict(postype='weibull'), **common_opts)
fig = probscale.probplot(x, ax=ax3, problabel='Custom plotting positions',
pp_kws=dict(alpha=0.6, beta=0.1), **common_opts)
ax1.set_xlim(left=1, right=99)
seaborn.despine()
fig.tight_layout()
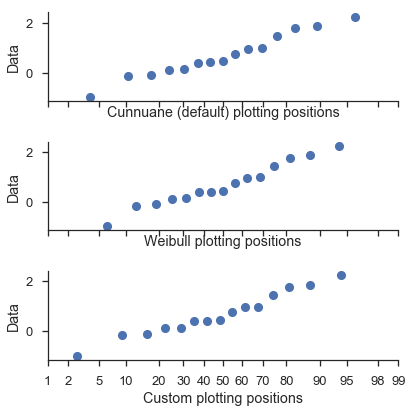
控制绘图元素的样式¶
正如上面的示例中提示的那样,probplot
函数接受两个字典来分别自定义数据系列和最佳拟合线(分别为 scatter_kws
和 line_kws
)。这些字典会直接传递到当前轴的 plot
方法。
默认情况下,数据系列假定 linestyle='none'
和 marker='o'
。可以通过 scatter_kws
覆盖这些值
回顾前面的示例,我们可以像这样自定义它
scatter_options = dict(
marker='^',
markerfacecolor='none',
markeredgecolor='firebrick',
markeredgewidth=1.25,
linestyle='none',
alpha=0.35,
zorder=5,
label='Meal Cost ($)'
)
line_options = dict(
dashes=(10,2,5,2,10,2),
color='0.25',
linewidth=3,
zorder=10,
label='Best-fit line'
)
fig, ax = pyplot.subplots(figsize=(4, 6))
fig = probscale.probplot(tips['total_bill'], ax=ax, plottype='prob', probax='y', bestfit=True,
datascale='log', problabel='Probabilities', datalabel='Total Bill (USD)',
scatter_kws=scatter_options, line_kws=line_options)
ax.legend(loc='upper left')
ax.set_ylim(bottom=0.1, top=99.9)
seaborn.despine()
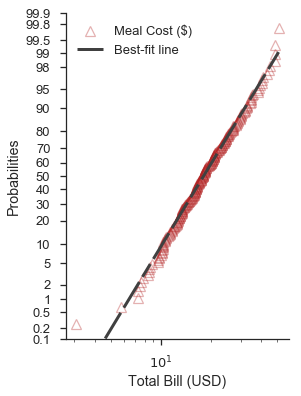
注意
probplot
函数可以接受两个额外的样式参数:color 和 label。如果提供,color 将覆盖 scatter_kws 和 line_kws 参数的标记面颜色和线条颜色选项。类似地,散点系列的标签将被显式参数覆盖。不建议使用 color 和 label。它们的存在主要是为了与 seaborn 包兼容。
将概率图映射到 seaborn FacetGrids¶
一般来说,probplot
的编写考虑到了 FacetGrids
。你只需在调用 FacetGrid.map
时指定数据列和其他选项。
不幸的是,标签的工作方式并不完全如我所愿,但这是正在进行的工作。
fg = (
seaborn.FacetGrid(data=iris, hue='species', aspect=2)
.map(probscale.probplot, 'sepal_length')
.set_axis_labels(x_var='Probability', y_var='Sepal Length')
.add_legend()
)
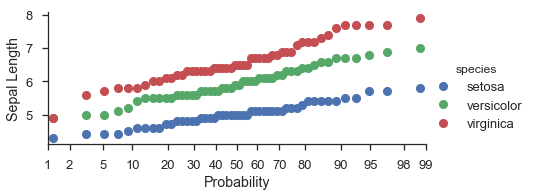
fg = (
seaborn.FacetGrid(data=iris, hue='species', aspect=2)
.map(probscale.probplot, 'petal_length', plottype='qq', probax='y')
.set_ylabels('Quantiles')
.add_legend()
)
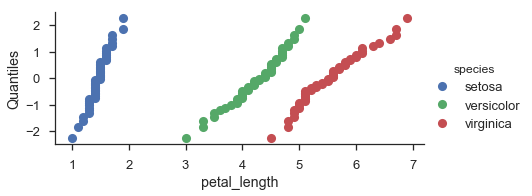
fg = (
seaborn.FacetGrid(data=tips, hue='sex', row='smoker', col='time', margin_titles=True, size=4)
.map(probscale.probplot, 'total_bill', probax='y', bestfit=True)
.set_ylabels('Probability')
.add_legend()
)
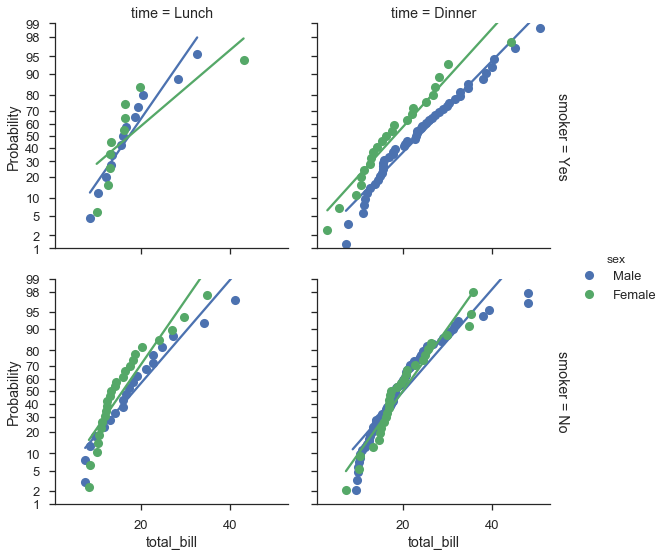